A recent look at the relationship between the NFL’s Wonderlic test and QB performance suggested that more intelligent QBs performed significantly better than less intelligent ones. Although it was a very interesting study it fell short in several respects.
The study found a very strong relationship between Wonderlic scores and total passing yards (and total TD passes) thrown within the first 4 years of a QB’s career. The study found that when only QBs with at least 1,000 yards total passing are included in the analysis, the results were very significant.
I thought a better way to the study would be to use a minimum number of pass attempts for inclusion, and to use career rate stats such as passing yards per attempt as the measure of performance. I also thought that some other passing stats such as completion percentage and interception rates may be particularly connected to intelligence. I re-did the study with these considerations in mind using the Wonderlic scores reported by the authors and gathering additional data from PFR.
I tested the correlations of various passing stats to Wonderlic scores using two different methods. The first method excluded QBs with fewer than 200 pass attempts and analyzed the remaining QBs (n=29). The alternate method included all the QBs with reported Wonderlic scores including those with very few attempts, but I replaced their stats with those of the 5th percentile qualifying QB (n=61). (My data file can be found here in case anyone wants to do his own analysis.)
This alternate method was intended to account for the fact that many QBs were not deemed good enough in the eyes of their coaches to start or play on a regular basis. Because I’m using rate stats for the analysis, “0.0 yards per attempt” is unrealistically low to assign to a QB. This methodology basically says that given the chance, those QBs would likely end up with replacement-level stats. I labeled this method “Replaced” in the table below.
The correlation coefficients between Wonderlic scores and various QB passing stats are listed below for both analysis methods. Keep in mind I’ve run several correlations, and we would expect to see an apparently significant correlation in some stats by chance 1 out of 20 times.
Stat | 200+ Att | Replaced |
Cmp% | -0.01 | -0.02 |
TD% | 0.03 | -0.08 |
Int% | 0.20 | 0.00 |
Yds/Att | -0.02 | -0.03 |
Adj Yds/Att | -0.10 | -0.04 |
QB Rating | -0.06 | -0.04 |
NY/A | 0.07 | 0.04 |
ANY/A | -0.03 | 0.01 |
Sk% | -0.23 | -0.13 |
Sk Yds/Att | -0.13 | -0.06 |
Adj Yds/Att = Adjusted Yds per Attempt (adjusted for interceptions and TDs)
NY/A = Net Yds per Attempt (yards per attempt factoring in sacks)
ANY/A = Adjusted Net Yds per Attempt (yards per attempt factoring in sacks, interceptions, and TDs)
The chart below is a scatterplot of Adjusted Net Yards per Attempt, a stat that considers nearly all aspects of QB passing performance.
These results tell a very different story than the strong correlations (r=0.50) found by the original authors. It appears that QB passing performance is not related to intelligence as tested by the Wonderlic. None of the correlations are statistically significant. The >200 attempts group requires a correlation of at least 0.31 to be significant, and the “Replaced” group requires at least a 0.21.
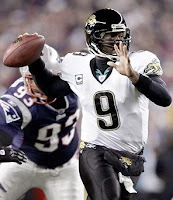
The study’s original authors concluded there was a strong connection between QB total passing yards and Wonderlic scores within the first 4 years of a QB’s career, but when we look at career rate stats, the relationship disappears. However, I think we shouldn’t overlook what this suggests.
Smarter QBs accumulate more total yards (and TDs) not because they turn out to be better players, but because they tend to start playing earlier in their careers. They may be able to learn the complex offensive systems of the NFL quicker, and their coaches would be more comfortable putting them behind center. Whether they are truly better prepared to play, or simply appear so to their coaches, would still be an open question.