It's 4th down and goal from the 2-yard line in the first quarter. What would most coaches do? Easy, they'd kick the field goal, a virtually certain 3 points.
But a 4th and goal from the 2 is successful about 3 out of 7 times, assuring the same number of expected points, on average, as the field goal. Plus, if the attempt at a touchdown is unsuccessful the opponent is left with the ball on the 2 or even 1 yard line. And if the field goal is successful, the opponent returns a kickoff which leaves them usually around the 28-yard line. It should be obvious that on balance, going for the touchdown is the better decision.
That's the case made by economist David Romer, author of a 2005 paper called "Do Firms Maximize, Evidence from Professional Football." Romer's paper is an analysis of 4th down situations in the NFL. It is quite possibly the most definitive proof that coaches are too timid on 4th down. Romer's theory is that coaches don't try to maximize their team's chances of winning games as much as they maximize their job security.
Coaches know that if they follow conventional wisdom and kick--oh well, the players just didn't make it happen. But if they take a risk and lose, even if it is on balance the better decision, they'll be Monday morning quarterbacked to death. Or at least their job security will be put in question.
In case anyone doubts how much coaches are concerned about Monday morning criticism, just take their word for it. Down by 3 points very late in the 4th quarter against the winless and fatigued Dolphin defense, former Ravens coach Brian Billick chose to kick a field goal on 4th and goal from one foot from the end zone. The Dolphins went on to score a touchdown in overtime. Billick's explanation at his Monday press conference was, "Had we done that [gone for it] after what we had done to get down there and [not scored a touchdown], I can imagine what the critique would have been today about the play call." Billick, a nine-year veteran head coach and Super Bowl winner, was more concerned about criticism from Baltimore Sun columnists than the actual outcome of the game. He'd rather escape criticism than give his team the best chance to win.
Romer's paper considers data from 3 years of games. To avoid the complications of particular "end-game" scenarios with time expiring in the 2nd or 4th quarters, he considers only plays from the 1st quarter of games. So his recommendations should be considered a general baseline for the typical drive, and not a prescription for every situation.
Romer's bottom line is the graph below. The x-axis is field position, and the y-axis is the yards-to-go on 4th down. The solid line represents when it is advisable for a team to attempt the first down rather than kick. According to the analysis, it's almost always worth it to go for it with less than 4 yards to go. The recommendation peaks at 4th and 10 from an opponent's 33 yard-line.
Romer basically measures the expected value of the next score. Say it's 4th and 2 from the 35 yd line. He compares the value of attempting a field goal from the 35 with the point value of a 1st and 10 from the 33 (multiplied the probability of actually making the first down.) He also recognizes that a field goal isn't always worth 3 points, and a touchdown isn't always worth at least 6. The ensuing kickoff gives an expected point value to the opponent. There is a point value to having a 1st and 10 from one's own 25 yard line.
One weakness of the paper is that it dismisses the concept of risk as unimportant. Romer says that long-term point optimization should be the only goal, so coaches should always be risk neutral. But if the level of risk aversion were actually considered, we might find that coaches are more rational than he concludes.
But the paper makes a very strong case that coaches should go for it on 4th down far more often than they currently do. Job security for coaches seems to be the primary reason why they don't. At a meeting with some researchers making the case for more aggressive 4th down decision making, Bengals coach Marvin Lewis responded, "You guys might very well be right that we're calling something too conservative in that situation. But what you don’t understand is that if I make a call that's viewed to be controversial by the fans and by the owner, and I fail, I lose my job."
It would be great if a coach came along and rarely kicked. It would be gamble, but if Romer and others are right, chances are the coach would be successful. And the rest of the NFL would have to adapt. It might only take one brave coach.
- Home Archives for February 2008
Going for It on Fourth Down
"Expert" Predictions
Gregg Easterbrook of ESPN.com writes a yearly column poking fun at all the terrible predictions from the previous NFL season. Here is his latest--It's long but highly entertaining. Unfortunately, it also makes a pretty good case that people like me with complicated mathematical models for predicting games are wasting our time. And the "experts" out there are doing even worse.
Predictions are Usually Terrible
His best line is "Just before the season starts, every sports page and sports-news outlet offers season predictions -- and hopes you don't copy them down." Unfortunately for them, he does.
Easterbrook's examples of horrible predictions underscores the fact that pre-season NFL predictions are completely worthless. Before the 2007 season I made the same point by showing that guessing an 8-8 record for every team is just as or more accurate than the "best" pre-season expert predictions or even the Vegas consensus. (Pay no attention to my own predictions attempt last June before I realized how futile it is.)
Unlike Easterbrook, most of us don't write our predictions down. It's easy to forget how wrong we were and how overconfident we were. So many of us go on making bold predictions every year.
Proof I'm (Almost) Wasting My Time
The most interesting part of the column might be the "Isaacson-Tarbell Algorithm." It's a system suggested by two of Easterbrook's readers last summer for predicting individual games. Just pick the team with the better record, and if both teams have the same record, pick the home team. According to Easterbrook, the Isaacson-Tarbell system would have been correct 67% of the time, about the same as the consensus Vegas favorites. Although devilishly simple, it requires no fancy computer models or expert knowledge and it would have beaten almost every human "expert" with a newspaper column, tv show, or website.
(Actually, I'm going to give credit for inventing the algorithm to my then 6-year old son who is an avid football fan (wonder why?). He devised that very same system during the 2006 season in a contest with my regression model and his grandfather in a weekly pick 'em contest. I'm sure many young fans have followed the same principle over the years.)
The model I built was accurate about 71% of the time last year. Is the extra 4% accuracy (10 games) worth all the trouble? Probably not (for a sane person) but I'll keep doing it anyway. Actually, I think 4% is better than it sounds. Why? Well, a monkey could be 50% correct correct, and a monkey who understood home field advantage could be 57% correct. It's a matter of how far above 57% can a prediction system get?
And there are upsets. No system, human or computer-based, could predict 100% accurately. They can only identify the correct favorite. Sometimes the better team loses. From my own and others' research, it looks like the best model could only be right about 75-80% of the time. So the real challenge is now "how far above 57% and how close to 80% can a system get?" There's only 23 percentage points of range between zero predictive ability and perfect predictive ability. Within that range, 4% is quite significant.
Better Ways to Grade Predictions
Phil Birnbaum of the Sabremetric Research blog makes the point that experts should not be evaluated on straight-up predictions but on predictions against the spread. I'm not sure that's a good idea, and I think I have a better suggestion.
Phil's point is that there are very few games in which a true expert would have enough insight to correctly pick against the consensus. Therefore, there aren't enough games to distinguish the real experts from the pretenders. His solution is to always pick against the spread.
I don't agree. The actual final point difference of a game has as much to do with the random circumstances of "trash time" as with any true difference in team ability. A better alternative may be to have experts weight their confidence in each game as way to compare their true knowledge.
Consider a hypothetical example Phil Birnbaum cited about an .800 team facing a .300. The true .800 team vs. true .300 team match-up is actually fairly rare. As Phil has eloquently pointed out previously, the .800 team may just be a .600 team that's been a little lucky, and the .300 team could really be a .500 team that's been a little unlucky. There are many more "true" .500 and .600 teams than .300 and .800 teams, so this kind of match-up is far more common than you'd expect. And if the ".500" team has home field advantage, we're really talking about a near 50/50 match-up. Although the apparent "0.800" team may still be the true favorite, a good expert can recognize games like this and set his confidence levels appropriately.
Computer Models vs. "Experts"
Game predictions are especially difficult early in the season, before we really know which teams are good. Over the past 2 years of running a prediction model, I've noticed that math-based prediction models (that account for opponent strength) do better than expert predictions in about weeks 3-8. The math models are free of the pre-season bias about how good teams "should" be. Teams like the Ravens and Bears, which won 13 games in 2006, were favored in games by experts far more than their early performance in 2007 warranted. Unbiased computer models could see just how bad they really would turn out to be.But later in the season, the human experts come around to realizing which teams are actually any good. The computer models and humans do about equally well at this point. Then when teams lose star players due to injury, the human experts can usually outdo the math models which have difficulty quantifying sudden discontinuities in performance.
And in the last couple weeks, when the best teams have sewn up playoff spots and rest their starters, or when the "prospect" 2nd string QB gets his chance to show what he can do for his 4-10 team, the human experts have a clear advantage. By the end of the season, the math models appear to do only slightly better than experts, but that's only really due to the particularities of NFL playoff seedings.
In Defense of Human Experts
Humans making predictions are often in contests with several others (like the ESPN experts). By picking the favorite in every game, you are guaranteed to come in first...over a several-year contest. But in a single-season contest, you'd be guaranteed to come in 2nd or 3rd to the guy that got a little lucky.
The best strategy is to selectively pick some upsets and hope to be that lucky guy. Plus, toward the end of the year, players that are several games behind are forced to aggressively pick more and more upsets hoping to catch up. Both of those factors have the effect of reducing the overall accuracy of the human experts. The comparison between math models and experts can often be unfair.
In Defense of Mathematical PredictionsLastly, in defense of the computer models, the vast majority of them aren't done well and give them a bad name. There is an enormous amount of data available on NFL teams, and people tend to take the kitchen-sink approach to prediction models. I started out doing that myself. But if you can identify what part of team performance is repeatable skill and what is due to randomness particular to non-repeating circumstances, you can build a very accurate model. I'm learning as I go along, and my model is already beating just about everything else. So I'm confident it can be even better next season.
Fumbles, Penalties, and Home Field Advantage
I had a theory that part of home field advantage may come from fumble recovery rates. Specifically, I was thinking of the kind of fumble that results in a pile of humanity fighting for the ball by doing things to each other only elsewhere done in prisons. It seems that the officials often have no better way of determining possession than by guessing which player has more control of the ball than the other guy. Sometimes it seems like they have a system--pulling the players off the pile one by one until they can see the ball. But in the end, they're still relying on their own judgment. There are complicating factors. Where was the ball when the play was whistled dead? When was the original ball carrier down? Was it a fumble or incomplete pass? In many cases, the process is analogous to basketball referees determining possession of a "jump ball" by their judgment of which player has better grip, or which player ultimately ripped the ball loose.
Perhaps the influence of the crowd had an effect on the officials by biasing their judgment. It's plausible because their have been many academic studies documenting the psychological effect of a home crowd on officiating in several sports. Much of the research focuses on penalties and fouls called by the officials, but what about other matters of judgment? Fumble recoveries might shed some light.
If the fumble recovery rate of home teams is significantly greater than away teams, then we'd have evidence that NFL officials are favoring home teams. The table below lists home and visiting team's fumbles and fumbles lost from the entire 2007 regular season encompassing 256 games.
Fumbles | Lost | Rate (%) | |
Visitor | 409 | 189 | 46.2 |
Home | 388 | 189 | 48.7 |
It appears that although visiting teams fumbled slightly more often, they lost possession less frequently. Neither difference is statistically significant, however, indicating that officials are unbiased in that department.
Although my fumble theory was a bust, what about penalties. Could the difference in penalties given to home and away teams be large enough to explain most of the home field advantage in the NFL? But if visiting teams in fact penalized more, it wouldn't necessarily indicate officiating bias. It could be due to crowd noise or other factors.
The table below lists The visitor and home penalty and penalty yard averages for the 2006 regular season.
Penalties/G | Pen Yards/G | |
Visitor | 6.2 | 50.1 |
Home | 5.8 | 48.1 |
I was very surprised by how small the difference is. On average, visiting teams only have 0.4 more penalties called (and accepted) on them than home teams for a difference of only 2 yards. I would expect the difference to be greater because of false start and delay of game penalties due to crowd noise.
In 2006, home teams won 55.6% of regular season games. According to the in-game model at Football Prediction Network, the difference of 2 penalty yards can only account for about 0.9% of the 5.6% home field advantage.
It appears that neither fumble recoveries nor penalties account for much of home field advantage in the NFL. Other factors such as travel fatigue or motivation are likely to be much more important. So I came up empty handed in the research...or so I thought until I came across some gems at Referee Chat Blog when doing some background research.
The author tracks officiating data from week to week, crew by crew. One of the most interesting things he's found is that crews don't tend to consistently favor home teams more than visiting teams across seasons (correlation = -0.04). Contrary to what was found in the study of officiating in British Premier League soccer I linked to above, NFL officials do not indicate a susceptibility to home crowd influence.
Many of the author's conclusions are based on differences in very small sample sizes (and he seems to realize this), but the data there are sound. Rex definitely knows his refs.
More Spygate Revelations
Without a doubt, the most popular and controversial article here at NFL Stats was one from last fall titled "Belichick Cheating Evidence?" Since then, there have been more revelations of rule-breaking, including the most recent allegations that the Patriots have been illegally taping signals since the 2000 season. Count me as one guy who is not surprised.
Back on September 15th, shortly after the League blew the whistle on the Patriots' signal taping, I wrote:
If Belichick's Patriots exploited unfair advantages in stealing signs from opposing sidelines we would expect to see some sort of evidence that they won games "beyond their means." By means I am referring to the Patriots' passing and running performance on offense and defense.
By successfully exploiting stolen signs, we might expect the Patriots to choose to use that advantage on critical plays--3rd downs in the 4th quarter for example. These critical plays would heavily "leverage" performance on the field to be converted into wins. In other words, the Patriots would win more games than their on field stats would indicate.
This is exactly what we see in the data. Year-in and year-out, Belichick's Patriots have won about 2 more games than expected given their offensive and defensive efficiencies, including turnovers and penalties. No other modern team has even come close to the Patriots in consistently winning more games than their stats indicate.
The model estimated how many wins a team would be expected to have each year based on its on-field abilities. The Patriots had won about 2 more games per year, every year, from 2002-2006 than their on-field performance would statistically indicate. In other words, other teams with similar performance stats win 2 fewer games in a season than Belichick's Patriots did. The graph below illustrates this trend.
My hunch is that not all of the over-performance is due to advantages gained from rule-breaking. I think the Patriots are focused intently on every last detail. Their scouting and research efforts are probably second to none. A team like that would squeeze every last advantage they could from every situation, and the taping was probably part of that larger effort which was partly legal and partly illegal.
2007 FG Kicker Ranking
There aren't many positions in team sports as lonely as the place kicker. Alone on the sidelines all game long, he's asked to make the game-winning field goal in overtime to send his team to the Super Bowl. Or maybe his head coach doesn't have enough faith in his leg to attempt a 48 yard try, and instead goes for it on 4th and 13 only to ultimately lose the Super Bowl by 3 points.
When we grade field goal kickers, we need to account for attempt distance and other factors. And attempt distance is complicated--it's non-linear. A 40 yard attempt is not twice as difficult as a 20 yd attempt. In fact, here is a graph of the average accuracy rates for field goals of various attempt distances.
So based on the analysis described here, I calculated the expected FG percentage for every NFL kicker based on his average attempt distance and home stadium environment. The difference between his actual FG% and his expected FG% can be considered his true performance given the difficulty of his attempts.
The table below lists all FG kickers from 2007 who had at least 10 attempts. It's sorted from best to worst. Click on the headers to resort as desired.
Kicker | Team | Avg Yds Att | # Att | Actual FG% | Exp FG% | Act-Exp % |
Feely | 36.6 | 23 | 91 | 83 | 9 | |
Bironas | 36.7 | 39 | 90 | 81 | 9 | |
Reed | 34.1 | 25 | 92 | 84 | 8 | |
Nedney | 36.1 | 19 | 89 | 81 | 8 | |
Graham | 33.4 | 34 | 91 | 85 | 6 | |
Longwell | 41.2 | 24 | 83 | 77 | 6 | |
Gould | 37.9 | 36 | 86 | 80 | 6 | |
Brown | 38.3 | 29 | 86 | 80 | 6 | |
Scobee | 33.5 | 13 | 92 | 86 | 6 | |
Lindell | 35.0 | 27 | 89 | 83 | 6 | |
Elam | 36.4 | 31 | 87 | 81 | 6 | |
Andersen | 34.3 | 28 | 89 | 84 | 5 | |
Kaeding | 35.1 | 27 | 89 | 84 | 5 | |
Kasay | 35.9 | 28 | 86 | 82 | 4 | |
Hanson | 38.7 | 35 | 83 | 80 | 3 | |
Dawson | 34.1 | 30 | 87 | 84 | 3 | |
Gostkowski | 32.9 | 24 | 88 | 85 | 2 | |
Brown | 37.5 | 34 | 82 | 80 | 2 | |
Suisham | 36.8 | 35 | 83 | 81 | 2 | |
Stover | 35.2 | 32 | 84 | 83 | 2 | |
Folk | 36.1 | 31 | 84 | 82 | 1 | |
Bryant | 35.0 | 33 | 85 | 84 | 0 | |
Tynes | 33.5 | 27 | 85 | 85 | 0 | |
Carney | 32.9 | 14 | 86 | 85 | 0 | |
Crosby | 38.2 | 39 | 79 | 80 | 0 | |
Nugent | 35.5 | 36 | 81 | 82 | -2 | |
Janikowski | 42.7 | 32 | 72 | 75 | -3 | |
Wilkins | 38.7 | 32 | 75 | 80 | -5 | |
Akers | 35.3 | 32 | 75 | 83 | -8 | |
Rackers | 39.1 | 30 | 70 | 79 | -9 | |
Vinatieri | 30.4 | 29 | 79 | 89 | -10 | |
Rayner | 38.0 | 22 | 68 | 80 | -11 | |
Mare | 34.7 | 19 | 53 | 84 | -31 |
Congratulations to Jay Feely and the Dolphins, who at least have bragging rights to something in 2007. And jeez, what happened to Mare down in New Orleans? Only 19 attempts, but still that's significantly bad to say the least. Rackers seemed to have a down year. He was one of the top FG kickers over the last two years.
(Except for Mare) kickers are mostly bunched together in performance. Although according to raw accuracy percentage they appear separated by a wide disparity, in reality the difference in performance among field goal kickers is not large. In my previous analysis, I estimated that one stadard deviation in true accuracy is 7.7%. And for every standard deviation difference, a kicker would yield on average an additional 2.3 field goals worth 6.7 points in a season. Whether that's considered a lot or not depends on your perspective.
Coaches and Risk
Recently I've been looking at risk and reward in the NFL using financial portfolio theory, a branch of math that analyzes and optimizes various risk-reward strategies. I've been building on previous research that applied the utility function to analyze each team's run/pass balance. In the last post, I calculated what each team's risk level (α) was for the 2006 season.
Risk was calculated as a level of risk aversion (or tolerance) based on the relative expected yardage gains and volatility of a team's running plays and passing plays. This method considers not only the simple ratio between run plays and pass plays, but the variance of each as well. For example, it considers whether a passing game is a short, high-percentage game or an aggressive down field game. Positive α means a team was risk averse, and negative α means a team was risk tolerant.
But grading play callers as risk tolerant or averse is slightly more complicated. I noted that winning teams were often the more conservative teams, but that conservative play calling was likely the result of having a lead. In other words, winning leads to conservative play calling, not the other way around.
I also noticed a clearly linear relationship between team wins and risk level. Below is a graph of risk aversion vs. team wins. We can see that teams with a lot of wins generally are the teams that can afford to be conservative.
The upward sloping line is the regression best-fit line. It suggests the typical level of risk for each number of season wins. For example, a team with 12 wins should have a fairly conservative profile, an α of about 0.02. And a team with 8 wins should have been more aggressive, with an α of about 0.01.
The distance above or below the best-fit line could be considered the excess risk beyond that which is appropriate for each number of wins. This value is the "residual" of the regression. Note that I said "could be considered." Keep in mind that an 8-win team that appears "too risky" may really be a 6-win team that gambled often and got lucky. There is an unquantified part of the equation that is random luck.
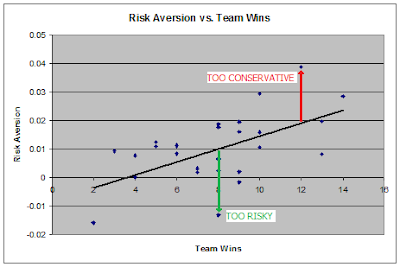
Now we have a way to score teams and coaches as risk averse or risk tolerant. The table below ranks each coach in terms of his excess risk, from the most risky to the most conservative. (I excluded Atlanta from the analysis because they were severe outliers in 2006. Vick's boom and bust scrambling style defied convention. The Falcons appeared to be over 20 times more aggressive than the next riskiest team due to their relatively very high variance in their running game.)
Team | Coach | Wins | Risk | Excess Risk |
Fisher | 8 | -0.013 | -0.0232 | |
Cowher | 8 | -0.011 | -0.0206 | |
Shanahan | 9 | -0.001 | -0.0137 | |
Smith | 13 | 0.008 | -0.0131 | |
Shell | 2 | -0.016 | -0.0122 | |
Holmgren | 9 | 0.002 | -0.0101 | |
Coughlin | 8 | 0.002 | -0.0075 | |
Nolan | 7 | 0.002 | -0.0059 | |
Jauron | 7 | 0.003 | -0.0046 | |
Reed | 10 | 0.011 | -0.0040 | |
McCarthy | 8 | 0.006 | -0.0037 | |
Fox | 8 | 0.007 | -0.0034 | |
Billick | 13 | 0.020 | -0.0016 | |
Del Rio | 8 | 0.009 | -0.0013 | |
Gruden | 4 | 0.000 | -0.0006 | |
Mangini | 10 | 0.016 | 0.0015 | |
Childress | 6 | 0.008 | 0.0030 | |
Edwards | 9 | 0.016 | 0.0039 | |
Shottenheimer | 14 | 0.029 | 0.0049 | |
Kubiak | 6 | 0.011 | 0.0057 | |
Saban | 6 | 0.011 | 0.0059 | |
Crennel | 4 | 0.008 | 0.0068 | |
Dungy | 12 | 0.026 | 0.0071 | |
Parcells | 9 | 0.019 | 0.0072 | |
Linehan | 8 | 0.018 | 0.0077 | |
Gibbs | 5 | 0.011 | 0.0078 | |
Lewis | 8 | 0.019 | 0.0090 | |
Green | 5 | 0.013 | 0.0094 | |
Marinelli | 3 | 0.009 | 0.0107 | |
Payton | 10 | 0.030 | 0.0151 | |
Belichick | 12 | 0.039 | 0.0197 | |
Mora | 7 | -0.438 | na |
Another way of looking at excess risk is presented in the graph below. The teams are sorted from most to fewest wins. Click to expand it.
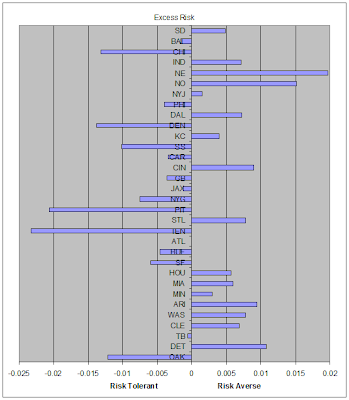
Of course we really could be talking about offensive coordinators rather than head coaches. But with few exceptions, it's the head coach that really sets his team's overall strategy. We're also only looking at one year--because it's the one year of data I have. It would be really interesting to see if some coaches consistently show the same level of risk aversion or tolerance over several seasons. But to get the data requires a play-by-play NFL database, something not readily available...yet.